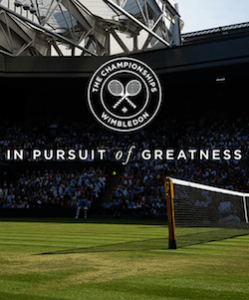
Wimbledon 2017 will be remembered for many things. Among others, this will include the first British woman, Johanna Konta, to make it to the semi-final since Virginia Wade in 1978. It marked the dramatic return of Ms Wimbledon, Venus Williams, 37, who reached her ninth SW19 final despite many heavy personal setbacks, only to be stonewalled by the 23-year-old Spaniard Muguruza. On the men’s side, the presence of Sam Querrey, an American, in the semi-final was the first since Andy Roddick in 2009. And then there was the magician, Roger Federer, 35, who became the oldest men’s finalist since the 39-year-old Ken Rosewall (in 1974), in his 11th final, taking his definitive place in the pantheon of the world’s greatest players, with his record 8th title in blistering form. And all that without losing a set.
Wimbledon Firsts…
It also marked a few firsts for the Wimbledon organisation itself. For example, it was the first time that Wimbledon provided highlight videos of the completed matches to the BBC with a completely automated service, powered by IBM’s Watson (artificial intelligence). Watson was capable of figuring out the best 2-4 minutes of each match on the show courts in a matter of moments and compiling and editing the video, keeping commentary and adding automatic text inserts. From the time of receiving the footage, the video creation time, according to IBM, was about 12 minutes. Over 250 videos were created in this manner over the two weeks.
I had the great privilege to be invited to go “under the hood” with IBM in their Wimbledon data dugouts. I could not help but find that, along with the performances by players like Williams and Federer in this tournament, Wimbledon is all about In Pursuit of Greatness.
Artificial Intelligence For Making Stories
How does Watson do these highlight videos? Essentially, to evaluate the raw footage, the system incorporates 6 major data points, two of which are human sources (courtside statistician and the referee’s office). The statisticians, who are all bona fide tennis players, mark down, rally by rally, the shots, direction, forced and unforced errors, etc. Two other elements come from the regular courtside monitor: serve speed data, player & ball position. Then comes the Cognitive Highlights input from Watson. Here, with a degree of deep learning, Watson normalises the on-court noise levels, then picks up on the crowd cheers and recognises the players’ actions (e.g. fist pumps, falls…).
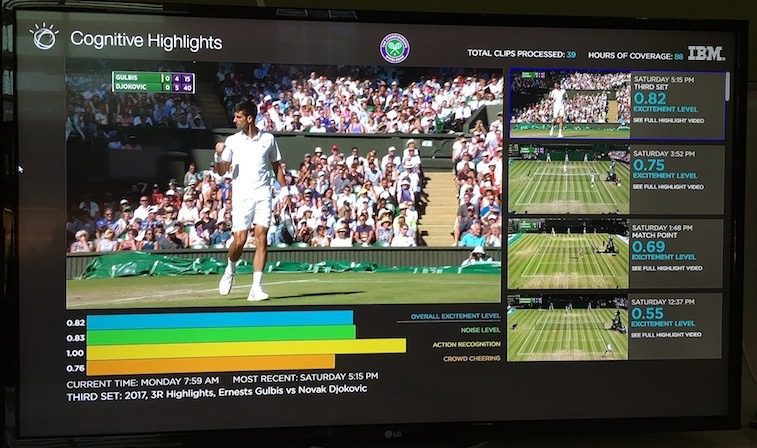
The AI kicks in to include the critical points. Thence, the system outputs a set of prioritised segments. The whole process is capped using metadata to create rather basic graphics that are layered on top. (You can read a more detailed description here from IBM’s Rogerio Feris).
Undoing Some Granny’s Tales — The Story of Data
Over the years of playing tennis, I have carried with me a number of principles that could be described variously as tennis lore, tennis truths and/or granny’s tales. I had three such principles.
- If you serve first, you are more likely to win. Well, the data paints a different figure than the granny’s tale. In 2017, the person serving first in the set won in 45% of the Gentlemen’s matches and 46% for the Ladies. For the whole period 2004-2016, the ratios are slightly higher: 47% and 50% respectively. It turns out that serving first in all sets after the first set is statistically a bad idea. Share on X Who would have known? See the revealing graphic created by IBM below.
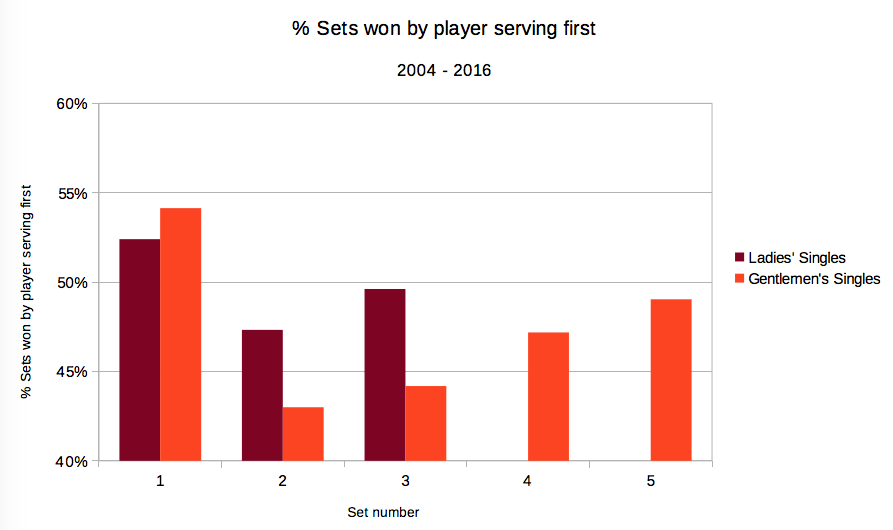
Data with Purpose
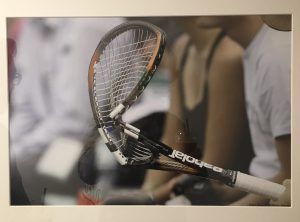
In my final chapter of data and tennis, I wished to explore how data could be used for good… to help make the world a better place! For example, I was wondering whether data could show that good manners, fair play and ethics might impact positively on the game and player results. From a fan perspective, there is no doubt about Federer’s legendary status thanks to his elegant play and graceful demeanour. But what kind of stats were there to support the benefits of being graceful, polite and fair? Could there be a link between passion and performance? For example: what might be the correlations between results with players that yelled, grunted too loud or smashed their racquet? There was no data to speak of … yet.
But, I did find one area that was scored: number of challenges and percentage of challenges won? What I found out was interesting, at least to me! First of all, the rates of winning challenges for men (25.5%) and women (29.6%) is low and reasonably close, albeit the women have the edge. Of the four finalists (women and men), Cilic and Berdych appeared in the top 20 with a respective (and respectable) 44.4% and 42.9% accuracy rate (winning 4/9 and 6/14 challenges). Of note, Cilic also made the largest number of challenges (14), equal with the up-and-coming Thiem. No sign of Muguruza, V Williams, Rybarikova, Konta, Querrey or even Federer among the winning challengers this tournament. Hum!
I’d love to see some data that help to support a cleaner, more sporting performance. As opposed to data for data’s sake, this would be data with purpose: data that shows a higher benefit than solely bigger winnings. Further, I’d like to show that being a bad sport is not good for tennis. That the circus of the server choosing the two best balls out of four almost-brand-new balls is quite unnecessary. And that superstitions are supercilious (e.g. touching nine different parts of your body before every serve is not effective). Stuff like that…
What do you think? How does this post make you react? Feel free to disagree or chime in!
It’s cool you got to see some of the IBM analytics process. I’ve been curious about it for a while. Several years ago, before matches, the commentators began talking about keys to the match. Often, they are something like, “If Williams can win 34% of her opponents 2nd serves, she has a 95% chance of winning.” That’s great and all, but it’s an ex post facto kind of of number that she has little actual input in making a reality. A better kind of metric they might put out is a correlation as follows: Williams wins 63% of points that are > 4 shots when she clears the net by 1.5 meters and her average spin rpm is >2300, this correlates to winning matches 80% of the time. 1) Williams can go into the match with an actual game plan. 2) Williams can also train to achieve these metrics, including using similar technologies used in tournaments (EVERY court at Indian Wells uses Shot Spot, while most outer courts in the Majors do not, which is f-ing pathetic, IMO!) like PlaySight.
I’ve written IBM and talked to several people about trying to get access to the Watson data, but it seems to be guarded and proprietary. I’d like to run my own numbers and look for patterns. For example, I suspect, through quantitative analysis, that many many unforced errors are made when simply trying to return a slice ground stroke. In my matches, generally tracing myself and my opponents, I notice a huge number of easy misses. This is useful data. There are myriad data analysis questions I have, and that would be fun points of conversation on TV tennis. It’d certainly be more useful than listening to John McEnroe compare everyone to himself, or Chris Evert talk about the courage of someone who hits a purely defensive lob on the full stretch that lands in a corner.
My dad and I were talking about the money issue. If IBM is selling the data, wow, a poor player could practically lose a match before even stepping onto the court with a player who can afford buying it and running infinite analysis of his/her game, the opponent’s game, and every shot in their head-to-head contests. I’d hate to see tennis cost barriers of entry climb even more simply based on analytics. As Joel said, wealthy players traveling with a private chef, physio, masseuse, full-time coach, etc. are already incredibly advantaged.
My sense is that IBM’s anticipation of statistics creates more crisp, professional viewership that is undetected and appreciate by many viewers. However, I like vinyl too and don’t mind hearing the hiss and crackle of an old record or an old broadcast.